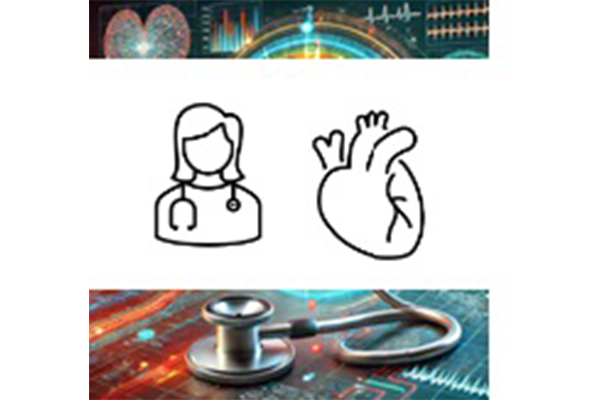
This research aims to enhance the diagnostic capabilities of electronic stethoscopes, making them effective tools for quantifying the severity of cardiovascular diseases (CVDs) at the point of care. Current stethoscope usage can only qualitatively identify potential CVDs, such as stenosis, but patients require expensive hospital-based tests to determine disease severity and guide treatment. The goal of this project is to create an affordable, accessible solution to improve healthcare in underserved regions, particularly in Africa.
The research will integrate machine learning with physics-based simulations of blood flow and the corresponding acoustic signals for different CVDs, focusing initially on supravalvular aortic stenosis (SVAS). The simulations will use 3D anatomically accurate models, potentially informed by medical imaging of patients. By classifying the acoustic signatures and blood-flow metrics from these simulations, machine learning will generate scaling laws to quantify the severity of CVDs. These models will be validated through in-vitro testing on a custom-built test rig.
Ultimately, the project aims to reduce the computational complexity required for electronic stethoscopes, allowing them to process patient data and accurately assess disease severity. The research will continue iteratively until the diagnostic accuracy of the system approaches 90% for a dataset of 1,000 patients.
Research areas
Image processing and pattern recognition with applications in medical imaging, Predictive analytics, Machine learning, Signal processing
Eligible researchers
Master's students, Post-master's students