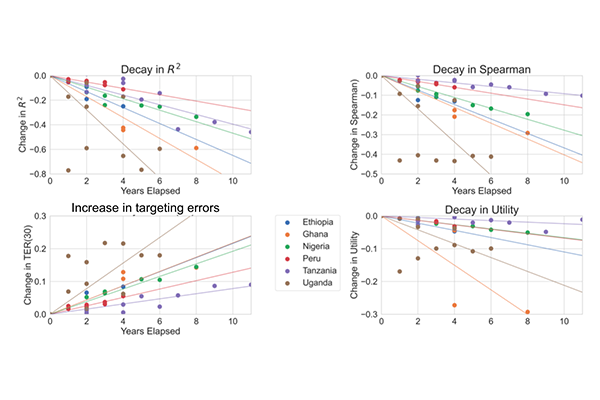
A key challenge in the design of effective social protection programs is determining who should be eligible for program benefits. In low and middle-income countries, one of the most common criteria is a Proxy Means Test (PMT) — a rudimentary application of machine learning that uses a short list of household characteristics to predict whether each household is poor, and therefore eligible, or non-poor, and therefore ineligible. However, there is an important weakness in this use of machine learning: the accuracy of the PMT prediction algorithm decreases steadily over time, as the model and underlying predictors grow out of date. Most PMTs used by governments are typically only updated every 5-10 years, implying that tens of millions of targeting errors occur globally due to this particular source of prediction error. This project will test whether methods from the ML robustness literature can improve the temporal robustness of a proxy means tests, reducing their tendency to decay in accuracy over time.
Research areas
Data science, Machine learning, Development
Eligible researchers
Master's students, Ph.D. students